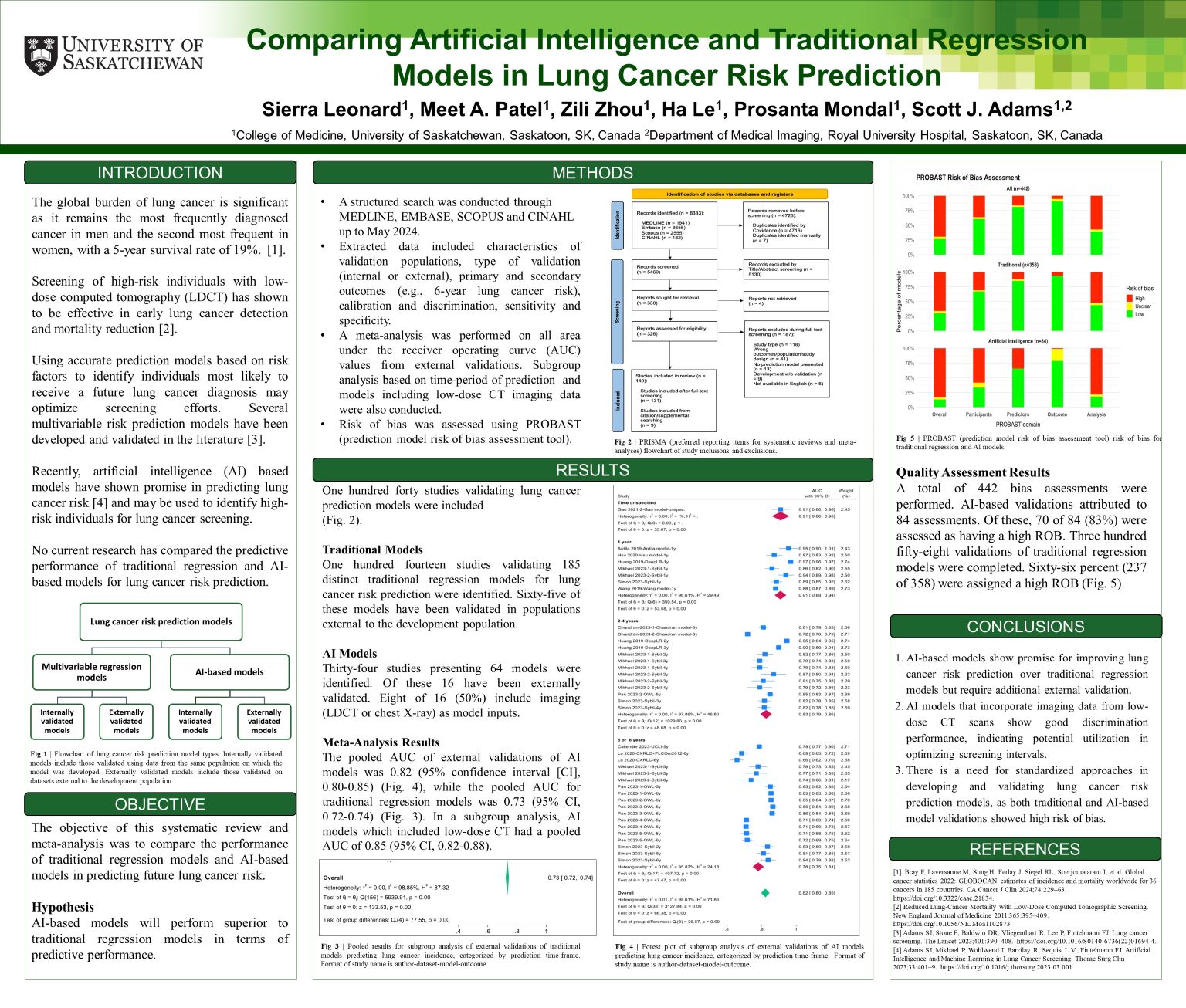
Comparing Artificial Intelligence and Traditional Regression Models in Lung Cancer Risk Prediction
Sierra Leonard
Purpose: To compare the performance of traditional regression models and artificial intelligence (AI)-based models in predicting future lung cancer risk.
Methods: A systematic review and meta-analysis were conducted with reporting according to PRISMA guidelines. We searched MEDLINE, Embase, Scopus, and CINAHL databases for studies reporting the performance of AI or traditional regression models for predicting lung cancer risk. Two researchers screened the articles and a third researcher resolved any conflicts. Model characteristics and predictive performance metrics were extracted. The quality of studies was assessed using the PROBAST tool. A meta-analysis was conducted to assess the discrimination performance of AI and traditional regression models, based on area under the receiver operating characteristic curve (AUC).
Results: One-hundred-forty studies met the inclusion criteria including 185 traditional regression and 64 AI-based models. The pooled AUC of external validations of AI models was 0.82 (95% confidence interval [CI], 0.80-0.85), while the pooled AUC for traditional regression models was 0.73 (95% CI, 0.72-0.74). In a subgroup analysis, AI models which included low-dose CT had a pooled AUC of 0.85 (95% CI, 0.82-0.88). Overall risk of bias was high for both AI and traditional models.
Conclusion: AI-based models, particularly those using imaging data, show promise for improving lung cancer risk prediction over traditional regression models, although the latter remain more extensively validated. Future research should focus on multi-center prospective validation of AI models and direct comparisons with traditional methods in diverse populations.