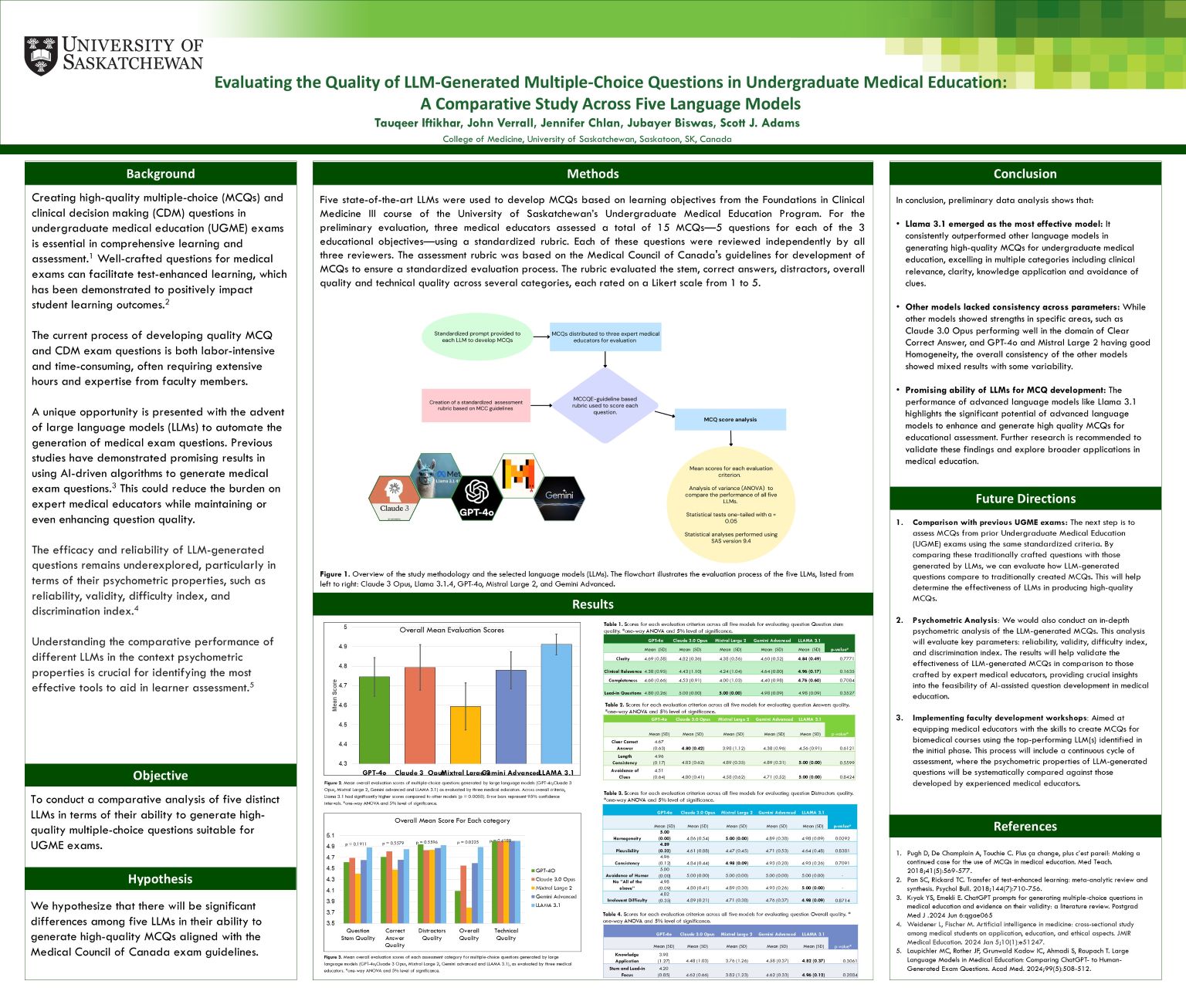
Evaluating the Quality of LLM-Generated Multiple-Choice Questions in Undergraduate Medical Education: A Comparative Study Across Five Language Models
Tauqeer Iftikhar
Objective: To conduct a comparative analysis of five distinct large language models (LLMs) in terms of their ability to generate high-quality multiple-choice questions (MCQs) suitable for Undergraduate Medical Education (UGME) exams.
Methods: Five state-of-the-art LLMs were used to develop MCQs based on learning objectives from the Foundations in Clinical Medicine III course of the University of Saskatchewan’s UGME Program. For the preliminary evaluation, three expert medical educators assessed a total of 15 MCQs using a standardized rubric. The rubric was developed using the Medical Council of Canada guidelines for developing MCQs. The rubric evaluated the stem, correct answers, distractors, overall quality, and technical quality across several categories, each rated on a Likert scale from 1 to 5.
Results: Llama 3.1 scored the highest in multiple domains, achieving the highest mean score of 4.91 (SD ±0.20; p = 0.006). While other models showed strengths in specific areas, such as Claude 3.0 Opus performing well in the domain of clear correct answer and GPT-4o and Mistral Large 2 performing well in the domain of homogeneity of distractors, the overall consistency of other models showed variability across generated MCQs.
Conclusion: The performance of advanced language models like Llama 3.1 highlights their significant potential to generate high quality MCQs for educational assessment. Further research is recommended to validate these findings and explore broader applications in medical education.